– Contributing to expand business opportunities of business operators by speeding up the service optimization process –
Researchers: O’UCHI Shin-ichi, Senior Researcher, Nano-CMOS Integration Group, Nanoelectronics Research Institute, and TAKANO Ryousei, Leader, Cyber Physical Cloud Research Group, Information Technology Research Institute
The researchers have developed a computing method and a circuit to speed up the training process of machine learning, and have demonstrated by simulation that the processing capacity for the training, which is currently difficult to speed up, can be increased by up to five-fold.
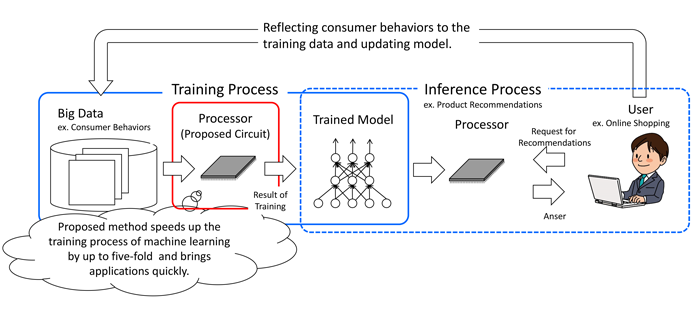 |
Figure 1. Process flow in machine learning and an example of application of the proposed computational method |
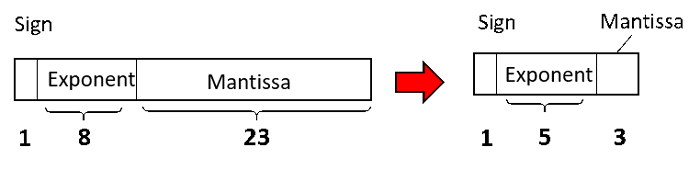 |
Figure 2. Data format: the conventional 32-bit format (left) and the proposed 9-bit format (right) |
Machine learning consists of inference and training processes. There is no definitive method for speeding up the training process. Problems faced include long processing time and high power consumption. In the training process of machine learning, there is a need for a data format that enables all data to be accurately represented by a limited number of bits and for a computational method and a circuit that enable multiplication and addition to be accurately performed with a small number of bits.
In the developed computational method, data consists of 1 bit for the sign, 5 bits for the exponent, and 3 bits for the mantissa. Multiplication is performed with 3 bits for the mantissa. Addition, which significantly affects computational accuracy, is performed by increasing the number of bits for the mantissa to 23. The researchers conducted a simulation using a circuit that enables this computational method to be implemented. The loss of computational accuracy was 2%. Since the circuit size can be reduced by about 80%, the power consumption was also reduced by 80%. It was confirmed that the training process can be sped up approximately five-fold.
To put the proposed computational method into practical use, the researchers will verify the effectiveness of the method by applying it to many more problems, and will prototype hardware to examine its feasibility.